A highly motivated and ambitious individual able to give timely and accurate advice, guidance, support and training to team members and individuals. Having the ability to work with the minimum of supervision whilst leading a team. Having a proven ability to lead by example, consistently hit targets, improves best practices and organizes time efficiently.
Wednesday, 10 December 2014
Eliminating Duplicates from the table
Source:
ROWID is the pseudo column,which is unique for every row.Based on this rowid the record can be eliminated.
Query:
DELETE FROM DUP_DEL
WHERE ROWID
NOT IN
(
SELECT MAX(ROWID) FROM DUP_DEL GROUP BY EMPNO
);
This calculates the maximum ROWID and deletes the rows which are not in the maximum ROWID.
After Delete:
ROWID is the pseudo column,which is unique for every row.Based on this rowid the record can be eliminated.
Query:
DELETE FROM DUP_DEL
WHERE ROWID
NOT IN
(
SELECT MAX(ROWID) FROM DUP_DEL GROUP BY EMPNO
);
This calculates the maximum ROWID and deletes the rows which are not in the maximum ROWID.
After Delete:
Friday, 5 September 2014
Factless Fact table
There are two types of factless fact tables: those that describe events, and those that describe conditions. Both may play important roles in your dimensional models.
Factless fact table for event or activity
When designing dimensional model, you often find that you want to track events or activities that occurs in your business process but you can’t find measures to track. In these situations, you can create a transaction-grained fact table that has no facts to describe that events or activities. Even though there are no facts storing in the fact table, the event can be counted to produce very meaningful process measurements.
Factless fact table for event or activity example
For example, you may want to track employee leaves. How often and why your employee leaves are very important for you to plan your daily activities and resources.
At the center of diagram below is the
FACT_LEAVE
table that has no facts at all. However theFACT_LEAVE
table is used to measure employee leave event when it occurs.
SELECT ENAME,COUNT(LEAVE_ID)
FROM FACT_LEAVE JOIN DIM_EMPLOYEE
ON FACT_LEAVE.EMPNO=DIM_EMPLOYEE.EMPNO
GROUP BY ENAME;
Factless fact table for condition, eligibility or coverage
Factless fact table can be also used in these situations:
- Tracking salesperson assigned to each prospect or customer
- Logging the eligibility of employees for a compensation program
- Capturing the promotion campaigns that are active at specific times such as holidays.
Those examples above describe conditions, eligibility or coverage. The factless fact table can be used to model conditions, eligibility or coverage. Typically information is captured by this star will not be studied alone but used with other business processes to produce meaningful information.
Let’s take a look at the sale star below. By looking only at the star, we don’t know what product has promotion that did not sell.
In order to track this kind of information, we can create a star that has factless fact table which is known as coverage table (according to Kimball).
In order to answer the question: what product that has promotion did not sell, we need to do as follows:
- Look at the second star to find out products that have promotions.
- Look at the first star to find out products that have promotion that sell.
- The difference between is the list of products that have promotion but did not sell.
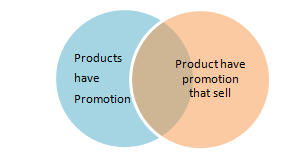
Factless fact table is crucial in many complex business processes. By applying concepts and techniques about factless fact table in this tutorial, you can design a dimensional model that has no clear facts to produce more meaningful information for your business processes.
Courtesy:zentut
Wednesday, 27 August 2014
Normalized Vs Dimensional Approach in DWH
The dimensional approach, made popular by in Ralph Kimball (website), states that the data warehouse should be modeled using a Dimensional Model (star schema or snowflake). The normalized approach, also called the 3NF model, made popular by Bill Inmon (website), states that the data warehouse should be modeled using an E-R model/normalized model.
In a dimensional approach, data is partitioned into either “facts”, which are generally numeric transaction data, or “dimensions“, which are the reference information that gives context to the facts. A key advantage of a dimensional approach is that the data warehouse is easier for the user to understand and to use. Also, the retrieval of data from the data warehouse tends to operate very quickly. The main disadvantage of the dimensional approach is that In order to maintain the integrity of facts and dimensions, loading the data warehouse with data from different operational systems is complicated. Plus, if you are used to working with a normalized approach, it can take a while to fully understand the dimensional approach and to become efficient in building one.
In the normalized approach, the data in the data warehouse are stored following database normalization rules. Tables are grouped together by subject areas that reflect general data categories (e.g., data on customers, products, finance, etc.). The normalized structure divides data into entities, which creates several tables in a relational database. When applied in large enterprises the result is dozens of tables that are linked together by a web of joins. Furthermore, each of the created entities is converted into separate physical tables when the database is implemented. The main advantage of this approach is that it is straightforward to add information into the database. A disadvantage of this approach is that, because of the number of tables involved, it can be difficult for users both to join data from different sources into meaningful information and then access the information without a precise understanding of the sources of data and of the data structure of the data warehouse.
In a dimensional approach, data is partitioned into either “facts”, which are generally numeric transaction data, or “dimensions“, which are the reference information that gives context to the facts. A key advantage of a dimensional approach is that the data warehouse is easier for the user to understand and to use. Also, the retrieval of data from the data warehouse tends to operate very quickly. The main disadvantage of the dimensional approach is that In order to maintain the integrity of facts and dimensions, loading the data warehouse with data from different operational systems is complicated. Plus, if you are used to working with a normalized approach, it can take a while to fully understand the dimensional approach and to become efficient in building one.
In the normalized approach, the data in the data warehouse are stored following database normalization rules. Tables are grouped together by subject areas that reflect general data categories (e.g., data on customers, products, finance, etc.). The normalized structure divides data into entities, which creates several tables in a relational database. When applied in large enterprises the result is dozens of tables that are linked together by a web of joins. Furthermore, each of the created entities is converted into separate physical tables when the database is implemented. The main advantage of this approach is that it is straightforward to add information into the database. A disadvantage of this approach is that, because of the number of tables involved, it can be difficult for users both to join data from different sources into meaningful information and then access the information without a precise understanding of the sources of data and of the data structure of the data warehouse.
Top-Down vs Bottom-Up Approach
Inmon’s top-down approach
Inmon defines data warehouse as a centralized repository for the entire enterprise. Data warehouse stores the ‘atomic’ data at the lowest level of detail. Dimensional data marts are created only after the complete data warehouse has been created. Thus, data warehouse is at the center of the Corporate Information Factory (CIF), which provides a logical framework for delivering business intelligence.
Inmon defines the data warehouse in the following terms:
- Subject-oriented: The data in the data warehouse is organized so that all the data elements relating to the same real-world event or object are linked together
- Time-variant: The changes to the data in the database are tracked and recorded so that reports can be produced showing changes over time
- Non-volatile: Data in the data warehouse is never over-written or deleted -- once committed, the data is static, read-only, and retained for future reporting
- Integrated: The database contains data from most or all of an organization's operational applications, and that this data is made consistent
Kimball’s bottom-up approach
Keeping in mind the most important business aspects or departments, data marts are created first. These provide a thin view into the organizational data, and as and when required these can be combined into a larger data warehouse. Kimball defines data warehouse as “A copy of transaction data specifically structured for query and analysis”.
Kimball’s data warehousing architecture is also known as Data Warehouse Bus (BUS). Dimensional modeling focuses on ease of end user accessibility and provides a high level of performance to the data warehouse.
Inmon vs. Kimball: Similar or different?
"You can catch all the minnows in the ocean and stack them together and they still do not make a whale." ~Inmon
“The data warehouse is nothing more than the union of all the data marts" ~Kimball
|
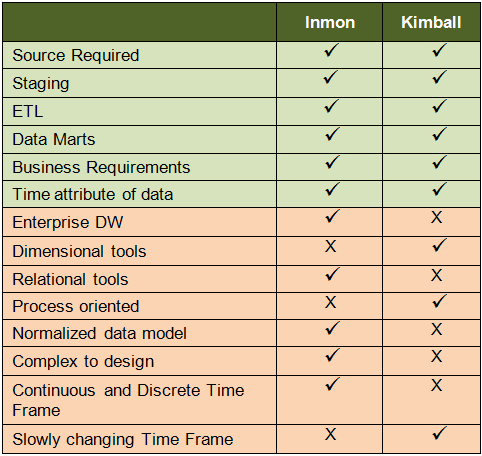
Pros and cons of both the approaches
Courtesy:Sansu George is a business analyst at ABIBA Systems
http://searchbusinessintelligence.techtarget.in/tip/Inmon-vs-Kimball-Which-approach-is-suitable-for-your-data-warehouse
Difference Between an ODS and a Datawarehouse
Answer1:
An ODS is a database that is subject-oriented, integrated, volatile and current. It is usually used by business managers, analysts or customer service representatives to monitor, manage and improve daily business processes and customer service. An ODS is often loaded daily or multiple times a day with data that represents the current state of operational systems.
An EDW is a database that is subject-oriented, integrated, non-volatile (read-only) and time-variant (historical). It is usually used by financial analysts for historical trend analysis reporting, data mining and other activities that need historical data. An EDW keeps growing as you add more historical snapshots, either daily, weekly or monthly. Because an EDW has historical data (and the ODS usually does not), some companies use the EDW as a hub for loading their data marts.
Answer2:
To a business user/information consumer, an ODS is best described as a means of moving the reporting function from the transactional systems so that users can report on current activity without impacting the performance of the transactional system. That is why the concept of an ODS was created. It is not a duplicate/copy of the OLTP system, but is designed to readily load transactions (DB logs) as well as facilitate creation of reports. The ODS may carry (store) data a little longer than the OLTP system, but generally it is defined as current/short term data. An ODS is normally tied to one OLTP system, so in a larger organization you may see multiple ODS instances.
ODS system generally works as a complementary system to EDW to achieve near real time analysis of important business facts. e.g. by end of every six hours, for a online marketing portal, it might be important to calculate total sales $ irrespective to whatever views (geography, marketing channels etc) sales has been done.
The DW on the other hand is the longer term repository of transactional data, and is designed around subject areas, rather than transactional applications. It normally has multiple systems sending data to it, and some of those systems can be ODS. It is designed to store data at the lowest level of detail (atomic) available from the data sources. The DW (or EDW) is the principle resource for providing normalized data to the business intelligence (BI) layer, either indirectly through a data mart/application or (infrequently) through direct query. Whether the DW design is 3NF or dimensional (federated data marts) probably wouldn't be of much concern to the end user. They just want a conformed, confident source of information without having to go to multiple sources to get it.
An ODS is a database that is subject-oriented, integrated, volatile and current. It is usually used by business managers, analysts or customer service representatives to monitor, manage and improve daily business processes and customer service. An ODS is often loaded daily or multiple times a day with data that represents the current state of operational systems.
An EDW is a database that is subject-oriented, integrated, non-volatile (read-only) and time-variant (historical). It is usually used by financial analysts for historical trend analysis reporting, data mining and other activities that need historical data. An EDW keeps growing as you add more historical snapshots, either daily, weekly or monthly. Because an EDW has historical data (and the ODS usually does not), some companies use the EDW as a hub for loading their data marts.
Answer2:
To a business user/information consumer, an ODS is best described as a means of moving the reporting function from the transactional systems so that users can report on current activity without impacting the performance of the transactional system. That is why the concept of an ODS was created. It is not a duplicate/copy of the OLTP system, but is designed to readily load transactions (DB logs) as well as facilitate creation of reports. The ODS may carry (store) data a little longer than the OLTP system, but generally it is defined as current/short term data. An ODS is normally tied to one OLTP system, so in a larger organization you may see multiple ODS instances.
ODS system generally works as a complementary system to EDW to achieve near real time analysis of important business facts. e.g. by end of every six hours, for a online marketing portal, it might be important to calculate total sales $ irrespective to whatever views (geography, marketing channels etc) sales has been done.
The DW on the other hand is the longer term repository of transactional data, and is designed around subject areas, rather than transactional applications. It normally has multiple systems sending data to it, and some of those systems can be ODS. It is designed to store data at the lowest level of detail (atomic) available from the data sources. The DW (or EDW) is the principle resource for providing normalized data to the business intelligence (BI) layer, either indirectly through a data mart/application or (infrequently) through direct query. Whether the DW design is 3NF or dimensional (federated data marts) probably wouldn't be of much concern to the end user. They just want a conformed, confident source of information without having to go to multiple sources to get it.
Characteristics of a Data warehouse
Data Warehouse characteristics set forth by Inmon:
Subject Oriented:Suppose you need to focus on your company's sales.So you better learn about company's sales data.Using this warehouse you can figure out "Who was the best customer for this year" or "Which item has been sold mostly this year"..Over all,Data warehouse in this case is defined by the matter of Subject.In this case,it is sales.
Integrated:Data warehouse is built from different source systems.Data should be maintained in a consistent format by eliminating all the discrepancies.Then it can be said as Integrated.
Non-Volatile:Once the data entered to the warehouse cannot be modified.Because that's what here to analyze the purpose.
Time Integrated:Analysts need a huge amount of data to identify the data patterns,business relationships.So it has to focus on change over time.
In general, fast query performance with high data throughput is the key to a successful data warehouse.
Subject Oriented:Suppose you need to focus on your company's sales.So you better learn about company's sales data.Using this warehouse you can figure out "Who was the best customer for this year" or "Which item has been sold mostly this year"..Over all,Data warehouse in this case is defined by the matter of Subject.In this case,it is sales.
Integrated:Data warehouse is built from different source systems.Data should be maintained in a consistent format by eliminating all the discrepancies.Then it can be said as Integrated.
Non-Volatile:Once the data entered to the warehouse cannot be modified.Because that's what here to analyze the purpose.
Time Integrated:Analysts need a huge amount of data to identify the data patterns,business relationships.So it has to focus on change over time.
In general, fast query performance with high data throughput is the key to a successful data warehouse.
Thursday, 31 July 2014
Dimensional and Relational: Not Opposites
A common misconception holds that the terms dimensional and relational are opposites. They are not.
The word "dimensional" describes a design method. The word "relational" describes a data storage technology.
As you can see from the diagram, a star schema is both relational and dimensional. So is a snowflake schema.
By the way, don't let this confuse you: most modern day DBMS products accommodate both kinds of storage.
The word "dimensional" describes a design method. The word "relational" describes a data storage technology.
- A dimensional model is a design approach that describes a process in terms of measurements (known as facts) and their context (dimensions)
- A star schema is a dimensional model implemented using relational storage technology—that is, in a relational database (RDBMS)
- A cube is a dimensional model implemented using multi-dimensional storage technology—that is, in a multidimensional database (MDB)
As you can see from the diagram, a star schema is both relational and dimensional. So is a snowflake schema.
By the way, don't let this confuse you: most modern day DBMS products accommodate both kinds of storage.
Monday, 28 July 2014
Is snapshot an aggregate
**This information is gathered from blogs of oaktonsoftware.It's purely for interesting reads and not of any commercial use.
Snapshot Fact Tables
A snapshot is a fact table that periodically samples a measurement. A snapshot always includes at least one measurement of status, such as a balance or level. A transaction fact table, in contrast, captures measurements as they occur.
The snapshot is useful for describing the effect of transactions, rather than transactions themselves. The best example is your bank account. Its all well and good if you have a record of each deposit, check, interest payment, fee, direct debit, and so forth. But what is your balance? Aggregating all your transactions is not the most expedient way to figure that out.
Sometimes Snapshots Summarize Transactions, but not Always
People sometimes think of snapshots as aggregates because they can be constructed from a table that contains transactions. That is certainly true in the case of account balances. If you have the transactions, you can compute the snapshots.
In this sense, you might be justified in thinking of a snapshot as an aggregate. But I don't. I'll explain why in just a second.
First, though, it is important to understand that not all snapshots are constructed from transactions. Sometimes, the number of transactions is too numerous to keep around. But in other cases,there simply aren't any. For example, we can't make use of transactions to describe the ambient temperature on the factory floor, or the level of pressure inside an industrial device. In these cases, the snapshot is clearly not a summary.
It really depends on how you define an aggregate.
The word aggregate is normally reserved for a summary table that does not transform the structure of its corresponding base table. For example, suppose account transactions are recorded in a fact table, with a fact called transaction_amount. A monthly aggregate would have exactly the same fact. Still called transaction_amount, is the same as the fact in the base table. The only difference is in level of detail: it represents a month's worth of transactions, rather than individual transactions. It is the same measurement.
Account balance (or other status measurements like levels) is a not the same as what the base fact table records. It is not an aggregation of transaction that occurred during the snapshot period; it is something more. It describes the net effect of the account transactions. It is a different measurement.
Conclusion
I use the term derived table to describe any table in a dimensional design uses another as its source. I save the word aggregate for non-transformative summaries.
To sum things up, then:
Yes, a snapshot sometimes summarizes a transaction fact table.
But if you accept these definitions for aggregate tables and derived tables, the answer is different:
A snapshot is is sometimes a derived table, but never an aggregate.
Snapshot Fact Tables
A snapshot is a fact table that periodically samples a measurement. A snapshot always includes at least one measurement of status, such as a balance or level. A transaction fact table, in contrast, captures measurements as they occur.
The snapshot is useful for describing the effect of transactions, rather than transactions themselves. The best example is your bank account. Its all well and good if you have a record of each deposit, check, interest payment, fee, direct debit, and so forth. But what is your balance? Aggregating all your transactions is not the most expedient way to figure that out.
Sometimes Snapshots Summarize Transactions, but not Always
People sometimes think of snapshots as aggregates because they can be constructed from a table that contains transactions. That is certainly true in the case of account balances. If you have the transactions, you can compute the snapshots.
In this sense, you might be justified in thinking of a snapshot as an aggregate. But I don't. I'll explain why in just a second.
First, though, it is important to understand that not all snapshots are constructed from transactions. Sometimes, the number of transactions is too numerous to keep around. But in other cases,there simply aren't any. For example, we can't make use of transactions to describe the ambient temperature on the factory floor, or the level of pressure inside an industrial device. In these cases, the snapshot is clearly not a summary.
It really depends on how you define an aggregate.
The word aggregate is normally reserved for a summary table that does not transform the structure of its corresponding base table. For example, suppose account transactions are recorded in a fact table, with a fact called transaction_amount. A monthly aggregate would have exactly the same fact. Still called transaction_amount, is the same as the fact in the base table. The only difference is in level of detail: it represents a month's worth of transactions, rather than individual transactions. It is the same measurement.
Account balance (or other status measurements like levels) is a not the same as what the base fact table records. It is not an aggregation of transaction that occurred during the snapshot period; it is something more. It describes the net effect of the account transactions. It is a different measurement.
Conclusion
I use the term derived table to describe any table in a dimensional design uses another as its source. I save the word aggregate for non-transformative summaries.
To sum things up, then:
Yes, a snapshot sometimes summarizes a transaction fact table.
But if you accept these definitions for aggregate tables and derived tables, the answer is different:
A snapshot is is sometimes a derived table, but never an aggregate.
Tuesday, 22 July 2014
Set Theory of JOINS
Those who are not familiar with the JOINS concept.Let's go back to the school.We had SET Theory in Mathematics.You might have heard like UNION,INTERSECT,MINUS.If you digest these things,JOINS is not a matter,but a syntax.
Let's Consider two sets,
A={1,2,3,4,5} B={1,3,5,7}
A UNION B ={1,2,3,4,5,7} --Just clubs all the elements without duplicates.
A UNION ALL B={1,1,2,3,3,4,5,5,7} ---Same as UNION,but with duplicates
A INTERSECT B={1,3,5} ----figures out only the common values in the sets.
A MINUS B={2,4} -- figures out the values which are common in A and B but not common in A.
B MINUS A={7} -- figures out the values which are common in A and B but not common in B.
Here,we have deptno as the common column between EMP and DEPT tables.Also,observe the distinct department numbers that the
tables have.
EMP table has {10,20,30,50}
DEPT table has {10,20,30,40}
If we apply the same mathematics here,then it really works.
UNION operator
SELECT DEPTNO FROM EMP
UNION
SELECT DEPTNO FROM DEPT;
Output:
10,20,30,40,50
UNION ALL operator
SELECT DEPTNO FROM EMP
UNION ALL
SELECT DEPTNO FROM DEPT;
Output:Unlike UNION,Combines all the departments without eliminating the duplicates.
INTERSECT operator
SELECT DEPTNO FROM EMP
INTERSECT
SELECT DEPTNO FROM DEPT;
Output:10,20,30
MINUS operator
SELECT DEPTNO FROM EMP
MINUS
SELECT DEPTNO FROM DEPT;
Output:50
SELECT DEPTNO FROM DEPT
MINUS
SELECT DEPTNO FROM EMP;
Output:40
Example:If you want to get the all the details of employees who has common departments between the tables.
INTERSECT vs INNER JOIN
SELECT * FROM EMP WHERE DEPTNO
IN
(
SELECT DEPTNO FROM EMP
INTERSECT
SELECT DEPTNO FROM DEPT
);
This is equivalent to INNER JOIN
SELECT * FROM EMP INNER JOIN DEPT ON EMP.DEPTNO=DEPTNO.DEPTNO;
INNER JOIN plays the role of INTERSECT
Like wise,
A LEFT OUTER JOIN B means
A INTERSECT B UNION A MINUS B
Let's Consider two sets,
A={1,2,3,4,5} B={1,3,5,7}
A UNION B ={1,2,3,4,5,7} --Just clubs all the elements without duplicates.
A UNION ALL B={1,1,2,3,3,4,5,5,7} ---Same as UNION,but with duplicates
A INTERSECT B={1,3,5} ----figures out only the common values in the sets.
A MINUS B={2,4} -- figures out the values which are common in A and B but not common in A.
B MINUS A={7} -- figures out the values which are common in A and B but not common in B.

tables have.
EMP table has {10,20,30,50}
DEPT table has {10,20,30,40}
If we apply the same mathematics here,then it really works.
UNION operator
SELECT DEPTNO FROM EMP
UNION
SELECT DEPTNO FROM DEPT;
Output:
10,20,30,40,50
UNION ALL operator
SELECT DEPTNO FROM EMP
UNION ALL
SELECT DEPTNO FROM DEPT;
Output:Unlike UNION,Combines all the departments without eliminating the duplicates.
INTERSECT operator
SELECT DEPTNO FROM EMP
INTERSECT
SELECT DEPTNO FROM DEPT;
Output:10,20,30
MINUS operator
SELECT DEPTNO FROM EMP
MINUS
SELECT DEPTNO FROM DEPT;
Output:50
SELECT DEPTNO FROM DEPT
MINUS
SELECT DEPTNO FROM EMP;
Output:40
Example:If you want to get the all the details of employees who has common departments between the tables.
INTERSECT vs INNER JOIN
SELECT * FROM EMP WHERE DEPTNO
IN
(
SELECT DEPTNO FROM EMP
INTERSECT
SELECT DEPTNO FROM DEPT
);
This is equivalent to INNER JOIN
SELECT * FROM EMP INNER JOIN DEPT ON EMP.DEPTNO=DEPTNO.DEPTNO;
INNER JOIN plays the role of INTERSECT
Like wise,
A LEFT OUTER JOIN B means
A INTERSECT B UNION A MINUS B
How to find second highest/lowest salary
Tuesday, 15 July 2014
FULL OUTER JOIN concept

SELECT A.ID,B.ID,C.ID
FROM A FULL OUTER JOIN B ON A.ID=B.ID
FULL OUTER JOIN C ON A.ID=C.ID OR B.ID=C.ID


It is easy only when you have understanding of JOINS concept in SQL.
Monday, 14 July 2014
Saturday, 12 July 2014
Count of NULL values in each column in a table
Display the count of all NULL values under each column in a table.
I have a table with random values ,but some of the values under each columns as NULL's.
Input: Output:
SELECT 'ID' AS COLNAME ,COUNT(*) FROM ABC WHERE ID IS NULL
UNION ALL
SELECT 'NAME' AS COLNAME ,COUNT(*) FROM ABC WHERE NAME IS NULL
UNION ALL
SELECT 'HIREDATE' AS COLNAME ,COUNT(*) FROM ABC WHERE HIREDATE IS NULL
Note:UNION ALL is used just to club the result fetched from each query.You can use UNION as well,but UNION checks for any duplicate rows.Since we know that there wont be any duplicate rows then we can use straight away UNION ALL in order to avoid the duplicate check.
AVG() function
Calculate the average commission without using AVG()
In general,we use
SELECT AVG(COMM) FROM EMP;
There is a chance to commit mistake here.Some people write
Here COUNT(*) gives 14 and the average value changes,which is incorrect.
RANK() versus DENSE RANK()
RANK() versus DENSE RANK()
Both of them are OLAP(Online Analytical Processing) functions.There is a difference between these two functions in assigning the ranks.
RANK function leaves the gaps between ranks when there is a same rank applied for multiple rows.
DENSE RANK functions applies the rank without leaving any gaps,though the same rank is applied for multiple rows.
SELECT EMPNO,ENAME,SAL,
RANK() OVER(ORDER BY SAL DESC) AS RANK,
DENSE_RANK() OVER(ORDER BY SAL DESC) AS DENSE_RANK
FROM EMP;
Note:The difference is precisely highlighted in the adjacent figure.
Same function can be applicable for Teradata database in TD14.
Find the repeated salaries
Display the details of the employees who are earning same salaries.
SELECT * FROM EMP WHERE SAL IN
(
SELECT SAL FROM EMP GROUP BY SAL HAVING COUNT(*)>1
);
--HAVING is used to filter the data only after GROUPing of data.
We cannot use the Aggregate functions in WHERE clause. eg: WHERE COUNT(*)=1 ,WHERE SUM(SAL)=1000 etc.
Multicolumn Subquery
Display the employee details who are earning maximum salaries of each department.
SELECT * FROM EMP WHERE(DEPNO,SAL) IN
(
SELECT DEPTNO,MAX(SAL) FROM EMP GROUP BY DEPTNO
);
Note:Here subquery plays an important role.
The columns in the subquery must be present in the WHERE clause of the outer query.
ACID Properties
ACID properties:
Atomicity: Transaction can be 0 or 1. All or None.
Eg: Suppose you are transferring funds from your account to another account,the transaction should be successful in such a way that the amount should be debited from your account and credited into another account.Then you can say the transaction is complete.It is not like the amount got debited from your account and not credited into another account or vice versa. In that case,the transaction gets rollback and transaction is unsuccessful.
Consistency:All the executed transactions data should be consistently maintained in the database.It's never left in half-finished state.
Isolation:All the transactions executes concurrently without any intervention of other transactions.
Durability: Database keeps track of pending changes in such a way that the server can recover from an abnormal termination.
Using SELF JOIN
Using SELF JOIN
Display the Employee Name and his Manager's Name from the below employee table:
Calculate TOP 3 salaries from employee table
Friday, 11 July 2014
Using GROUP BY,HAVING,DISTINCT
Using GROUP BY,HAVING,DISTINCT
I need to get the employees who owns multiple cars and multiple distinct cars.
The above data says the cars owned by the employees in a company.An employee has multiple cars.It can be same car or different car.
Multiple Cars:
SELECT ID FROM CARS GROUP BY ID HAVING COUNT(*)>1;
--Gives 2,4,5,6
Multiple Distinct Cars:
SELECT ID FROM CARS GROUP BY ID HAVING COUNT(DISTINCT CAR)>1;
--Gives 2 and 4
Subscribe to:
Posts (Atom)